Big Deta Analytics
HYPERGRID Technology Sloutions Big Data and Data Analytics Services encompass a range of solutions to help organizations collect, store, process, and analyze vast amounts of data. These services provide the expertise and infrastructure needed to transform raw data into actionable insights, enabling businesses to make data-driven decisions, optimize operations, and stay competitive.
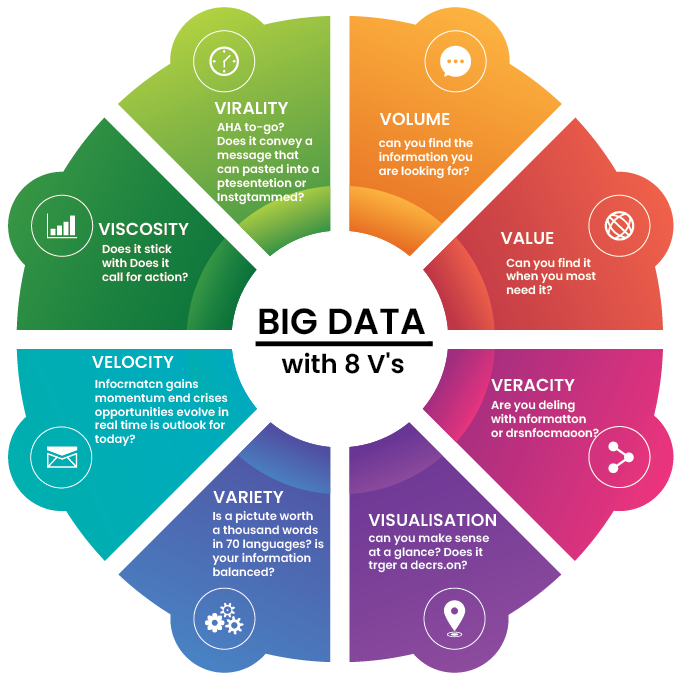
Here’s an overview of the Big Data and Data Analytics Services typically offered:
1. Data Strategy and Consulting
2. Data Engineering and Integration
3. Big Data Storage and Management
4. Data Analytics and Visualization
5. Machine Learning and Artificial Intelligence (AI)
6. Advanced Analytics and Business Intelligence (BI)
7. Big Data Infrastructure Setup and Management
8. Data Science as a Service (DSaaS)
9. Data Quality and Data Cleaning
10. Big Data Managed Services
Benefits of Big Data and Data Analytics Services:
Big Data and Data Analytics Services empower organizations to harness the power of data for transformative insights and sustained competitive advantage. Whether through consulting, data engineering, or advanced analytics, these services help turn data into a strategic asset.